How UCHealth is reducing fall injuries with AI-enhanced risk modeling
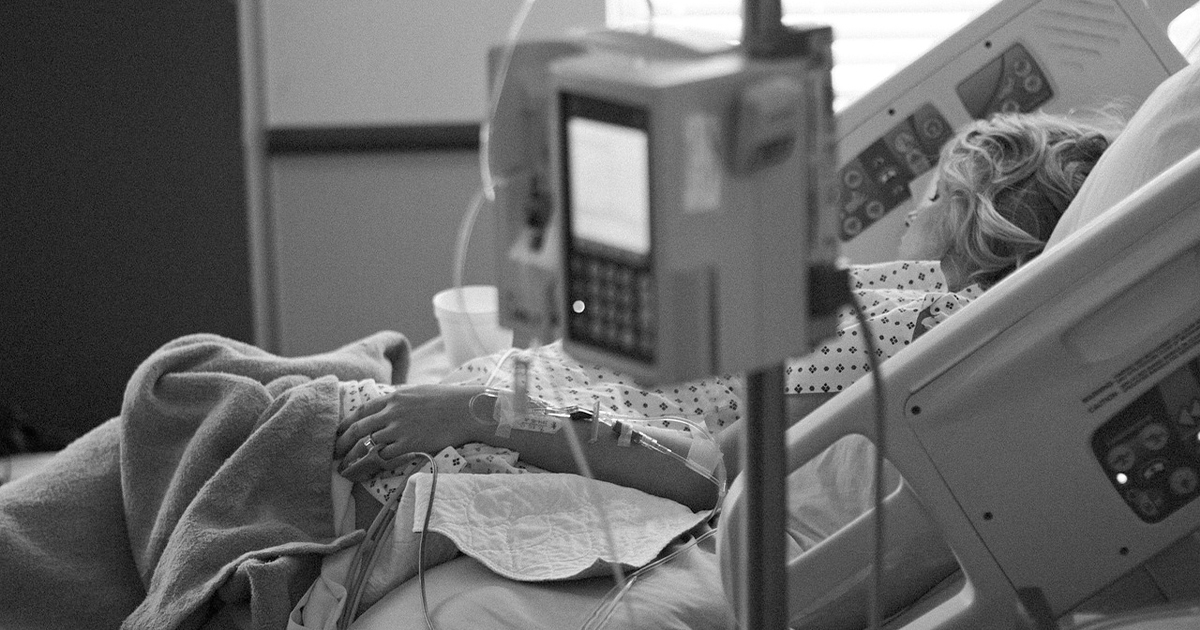
Image: Parentingupstream/Pixabay
Inpatient clinical care teams generally use tools such as bed alarms, gait belts, closer nursing station placement and more to prevent patient falls.
But those interventions can hinder clinical workflows and alert fatigue can make them less effective than they're meant to be.
To help ensure only high-risk patients receive intensive precautions, and clinicians' alarm fatigue is reduced, Aurora, Colorado-based UCHealth has developed a novel user interface that serves up specific fall interventions based on a patient’s unique risk profile.
The tool uses mobility data, behavioral health indicators and other risk factors to predict the risk of inpatient falls with injury and leverages cloud tools to integrate the predictions right into the Epic electronic health records.
The strategy takes "a great deal of data being entered into the EHR in other work streams and clearing out the noise to bring the clinician a clear recommendation," said Brendan Drew, a data scientist focusing on UCHealth's inpatient operations, and Brittany Cyriacks, a clinical informaticist at the health system.
We caught up with Drew and Cyriacks about their efforts to leverage artificial intelligence and cloud tools. They'll explain more during an education session at HIMSS25 in Las Vegas next month.
Q. How was the model created, and what are its key objectives?
A. Our multidisciplinary team conducted a literature review that identified risk variables – 12 risk domains, 92 potential variables – and mapped them to Epic data elements. They sampled data from more than 181,000 inpatient admissions, including more than 200 features per admission.
Initially, both XGBoost and a regularized logistic regression model were tested. Ultimately, top features were re-engineered and combined into a simplified logistic regression model.
The model runs every four hours, ingesting the most recent clinical documentation. Rather than a simple high/low indicator, a three-tiered classification – Highest Risk, Elevated Risk and Universal Risk – gives more clinically actionable results.
Classification generates a list of risk-level and patient-specific precautions that can keep patients safe.
Q. What were some challenges of integrating the model into clinical workflows?
A. The team needed to define clear, tiered precautions (e.g., 'Highest Risk' patients should have chair/bed alarms, be kept within arm’s reach, etc.) so staff knew exactly what actions to take at each risk level.
In addition to standardizing precautions, the presentation will also highlight the following key challenges:
- Meaningful display of risk: Merely showing a numeric score was not sufficient. Clinicians needed to see why a patient was flagged as high risk and which precautions were recommended.
- Data availability in the first 12 hours: The model requires sufficient clinical data entry and existence. During the first 12 hours, risk categorizations could be incomplete, so staff must exercise clinical judgment to decide if the patient would benefit from implementing universal precautions or additional precautions without relying solely on the model.
- User trust and adoption: Ensuring nurses and other care team members trusted the AI recommendations was critical. Ongoing communication, training and a user-friendly interface helped build confidence. Data surrounding fall events has helped drive the conversation away from trust in the AI recommendation and more towards barriers to implementing precautions for the patient.
- Workflow integration: Rather than adding an extra step for clinicians, the model’s outputs and recommended precautions were built into the existing Epic flowsheets, pop-ups and care plans so staff could use them naturally.
Q. Will the session offer insights into lessons learned using AI to reduce fall injury risks?
A. Yes. The presentation includes strategies for the successful adoption of predictive models, measuring model performance, addressing disparities among different populations (Spanish-speaking patients, for example) and ensuring continuous improvement.
It also highlights real-world 'what we have learned so far' themes, offering attendees practical takeaways on how to deploy, monitor and refine AI tools for fall prevention. We will emphasize the engagement that is needed by the organization and nurses along the way.
Drew and Cyriacks' session, "Fall Injury Risk Model: From AI to Clinical Interventions," is scheduled for Tuesday, March 4, from 3:15-4:15 p.m. at HIMSS25.